Rudy Lai ยท June 12, 2021
Finding key challenges at 1000 companies in 1 day
As the cost of product development gets cheaper, markets get more crowded, more complicated, and more competitive. At the same time, everybody is now enabled by automated sales tools that generate a huge amount of noise.
Taking time to understand your customer to personalise your approach becomes more and more important.
The keyword here is time.
It's all well and good to say that "we need to focus on personalisation", or "we need to make sure that everything is personalised", but often there is just not enough hours in a day to find the right balance between quality and quantity.
Here's one way we have solved this at Tactic.
What we are doing
We built 5 sequences that target the top 5 common pain points that our solution solves. We go through 200-300 pieces of content (webpages, articles, PDFs, job posts etc.) per company to understand whether they suffer from that pain. If they do, we assign them to one of the 5 sequences, and repeat.
Doesn't that take more time?
I hear you already. "go through 200-300 articles? Doesn't that take all day?"
It doesn't, because we use AI to summarise these articles and highlight indicators of the pain points we solve, and only look at this company if there is anything in the summary. If the summary is empty, we know that no pain exists and we can move on.
Reading the summary takes just a minute.
Let's go through how this works.
Step 1: Create the sequences
We built 5 sequences that target the top 5 common data problems that our solution solves. By looking at our value matrix (personas mapped to goals and pain), we have selected:
- time spent qualifying accounts
- time spent finding triggers for personalisation
- coaching a lot of new reps on a complex product
- developing and implementing outbound for multiple regions and verticals
- obtaining high quality and trustworthy data for territory management
We create a sequence targeting each pain point, with a simple framework of problem + case study. This helps the buyer understand their problem better and see how we helped a company in their industry solve that problem.
The message itself only personalises on name and company, but it works because we have done the research to ensure that we only target companies with that pain point recently.
Here's an example template for pain point 1:
Hey {{firstname}}, I can see that {{company}} focuses on driving your sales team towards the right activities using data. I also noticed that a large % of your headcount is in the sales team.
Working with sales leaders in similar situations, a common data problem is to find sources for all the data points you need for your ICP. You buy the major data points, like industry, software usage, and funding, but your team still spends 6-8 hours a week going through other data to find high fit accounts.
Is that a familiar challenge?
Step 2: List out pain point indicators + data behind the message
There is almost an infinite amount of data at our disposal. What do we focus on?
Let's take pain point #1. I am sure nobody comes out and say "hey we are wasting time qualifying accounts, help us!" (although I have seen this before, usually in a very candid podcast)
Instead, we can look at indicators:
- large sales team relative to company size
- mentions of 'data governance', 'data quality' etc in a sales context
- sales leaders talking about data
- execs focused on sales productivity
to name a few.
Step 3: Use AI to gather and summarise data
In a day, we gather data across 1,000 companies, highlight key information using people and keyword searches, and distill that into a summary. Here's how we map the indicators we have discussed into searches:
- people search for "account executive", "sales/business development", and "sales/revenue operations"
- keyword searches like 'data governance', 'data quality' etc.
- keyword searches linking "CRO" to "data" etc.
- keyword searches for any mentions of "sales productivity", "productivity per rep" etc.
Step 4: Review summaries and tag companies
After the summaries are generated, we have to review them manually. Our sales team goes through this process.
As mentioned before, if a summary is empty, we can be sure that there isn't relevant pain in that company.
We rank the companies by how much information there are in the summary, and then make a decision based on the indicators:
- do they have a large sales team relative to the company?
- are they thinking about 'data governance', 'data quality' etc in a sales context, which we can also use to design our campaign?
- are their sales leaders talking about data?
- what's their sales productivity metrics looking like? are they performing?
Based on this information, we can assign them to 1 of 6 labels - most commonly, we bucket it into 'no pain', and then followed by one of the 5 pain points we commonly solve.
Step 5: Launch!
After we do this for 1000 companies, we usually end up with 150-200 companies that may have a challenge we can solve. We then reach out to 10 people per company across our different personas, with the same template. We do this over a 2 week period.
We also participate in the conversation on LinkedIn with these folks, usually uncovering even more initiatives and challenges.
By the numbers:
- Time spent - 1 day waiting for the data processing, 2 days going over the results and assigning companies to the right sequences
- Time saved - let's say it takes you 30 minutes to manually research an account. 1000 companies worth of research x 30 minutes = 500 hours = 21 days. So we saved 21 days - 3 days = 18 days in total.
- Companies prospected - 1,000
- Companies reached - 200
- People reached - 2,000
We'd love to hear from you if you gave this a try and saw success!
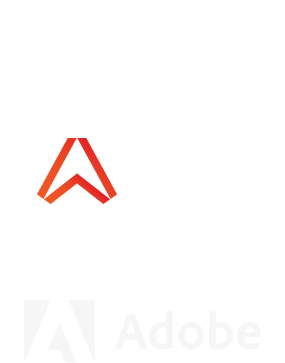